Many of the protein shapes are from organisms that are completely unknown to science.
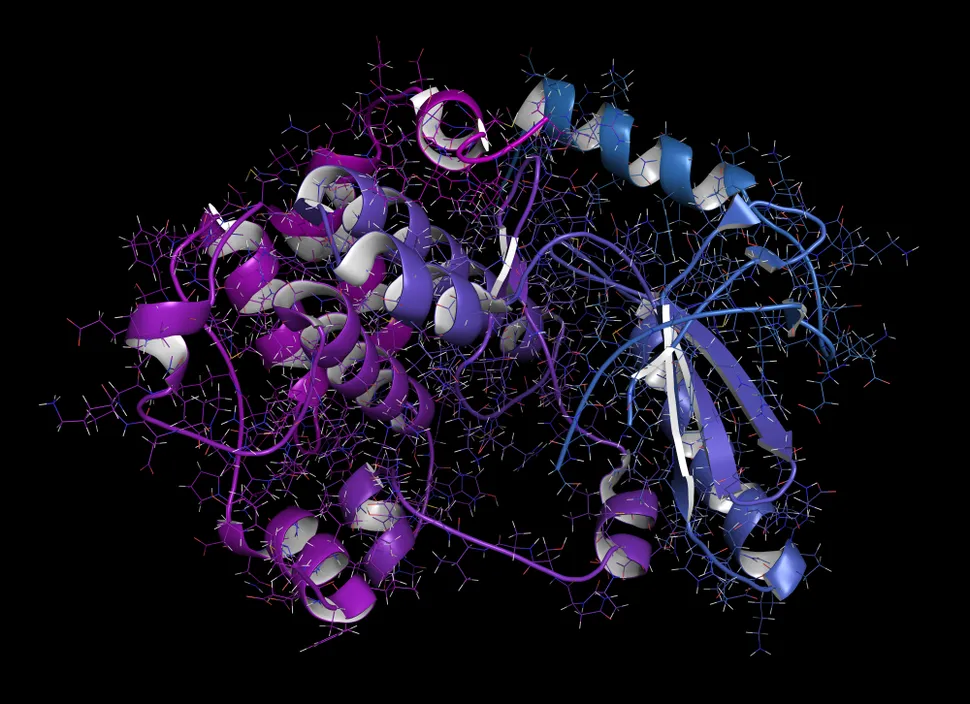
Scientists at Meta, the parent company of Facebook and Instagram, have used an artificial intelligence (AI) language model to predict the unknown structures of more than 600 million proteins belonging to viruses, bacteria and other microbes.
The program, called ESMFold, used a model that was originally designed for decoding human languages to make accurate predictions of the twists and turns taken by proteins that determine their 3D structure. The predictions, which were compiled into the open-source ESM Metagenomic Atlas, could be used to help develop new drugs, characterize unknown microbial functions, and trace the evolutionary connections between distantly related species.
ESMFold is not the first program to make protein predictions. In 2022, the Google-owned company DeepMind announced that its protein-predicting program AlphaFold had deciphered the shapes of the roughly 200 million proteins known to science. ESMFold isn’t as accurate as AlphaFold, but it’s 60 times faster than DeepMind’s program, Meta says. The results have not yet been peer-reviewed.
“The ESM Metagenomic Atlas will enable scientists to search and analyze the structures of metagenomic proteins at the scale of hundreds of millions of proteins,” the Meta research team wrote in a blog post accompanying the release of the paper to the preprint database bioRxiv. “This can help researchers to identify structures that have not been characterized before, search for distant evolutionary relationships, and discover new proteins that can be useful in medicine and other applications.”
Proteins are the building blocks of all living things and are made up of long, winding chains of amino acids — tiny molecular units that snap together in myriad combinations to form the protein’s 3D shape.
Knowing a protein’s shape is the best way to understand its function, but there are a staggering number of ways the same combination of amino acids in different sequences can take shape. Despite proteins quickly and reliably taking certain shapes once they’ve been produced, the number of possible configurations is roughly 10^300. The gold standard way to determine a protein’s structure is using X-ray crystallography — seeing how high-energy light beams diffract around proteins —, but this is a painstaking method that can take months or years to produce results, and it doesn’t work for all protein types. After decades of work, more than 100,000 protein structures have been deciphered via X-ray crystallography.
To find a way around this problem, the Meta researchers turned to a sophisticated computer model designed to decode and make predictions about human languages, and applied the model instead to the language of protein sequences.
“Using a form of self-supervised learning known as masked language modeling, we trained a language model on the sequences of millions of natural proteins,” the researchers wrote. “With this approach, the model must correctly fill in the blanks in a passage of text, such as “To __ or not to __, that is the ________.” We trained a language model to fill in the blanks in a protein sequence, like “GL_KKE_AHY_G” across millions of diverse proteins. We found that information about the structure and function of proteins emerges from this training.”
To test their model, the scientists turned to a database of metagenomic DNA (so named because it has been sequenced in bulk from environmental or clinical sources) taken from places as diverse as soil, seawater and the human gut and skin. By feeding the DNA data into the ESMFold program, the researchers predicted the structures of over 617 million proteins in just two weeks.
That’s over 400 million more than AlphaFold announced it had deciphered four months ago, when it claimed to have deduced the protein structure of almost every known protein. This means that many of these proteins have never been seen before, likely because they come from unknown organisms. More than 200 million of ESMFold’s protein predictions are thought to be high-quality, according to the model, meaning that the program has been able to predict the shapes with an accuracy down to the level of atoms.
The researchers are hoping to use this program for more protein-focused work. “To extend this work even further, we’re studying how language models can be used to design new proteins and contribute to solving challenges in health, disease, and the environment,” Meta wrote.
Add Comment