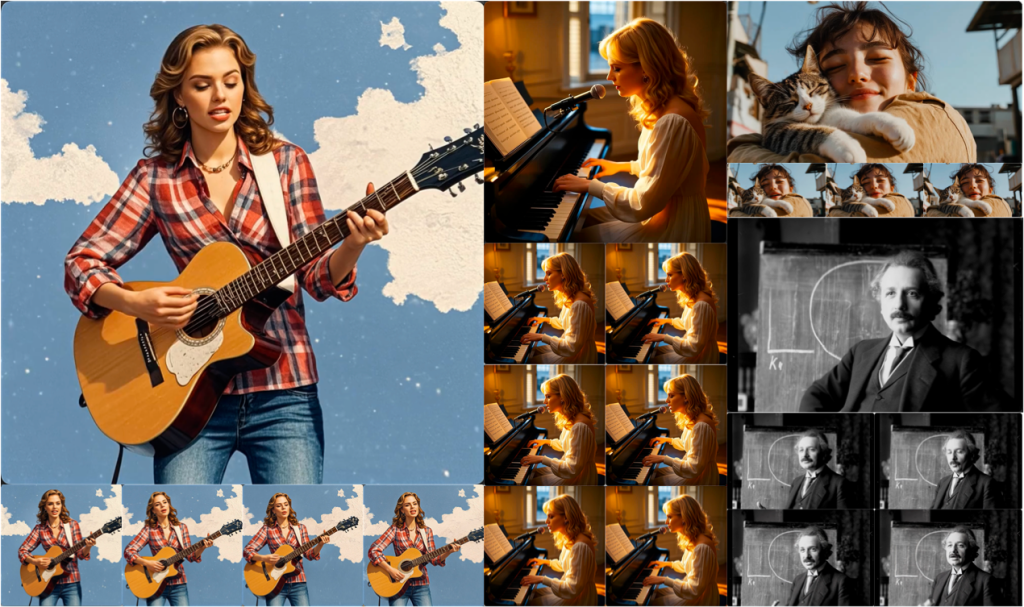
movements, as well as facial expressions, that match the audio. OmniHuman generates highly realistic videos with any aspect ratio and body
proportion, and significantly improves gesture generation and object interaction over existing methods, due to the data scaling up enabled by
omni-conditions training.
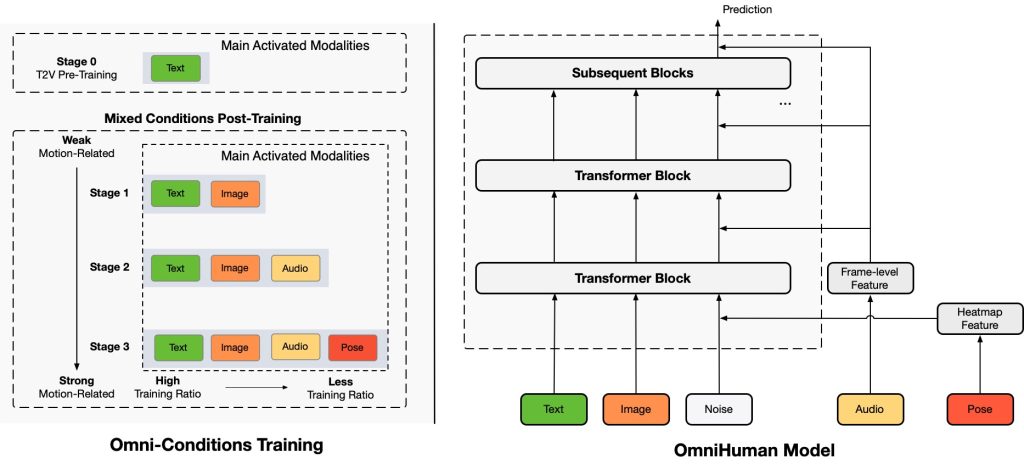
and supports simultaneous conditioning with multiple modalities including text, image, audio, and pose; (2) the omni-conditions training
strategy, which employs progressive, multi-stage training based on the motion-related extent of the conditions. The mixed condition training
allows the OmniHuman model to benefit from the scaling up of mixed data.
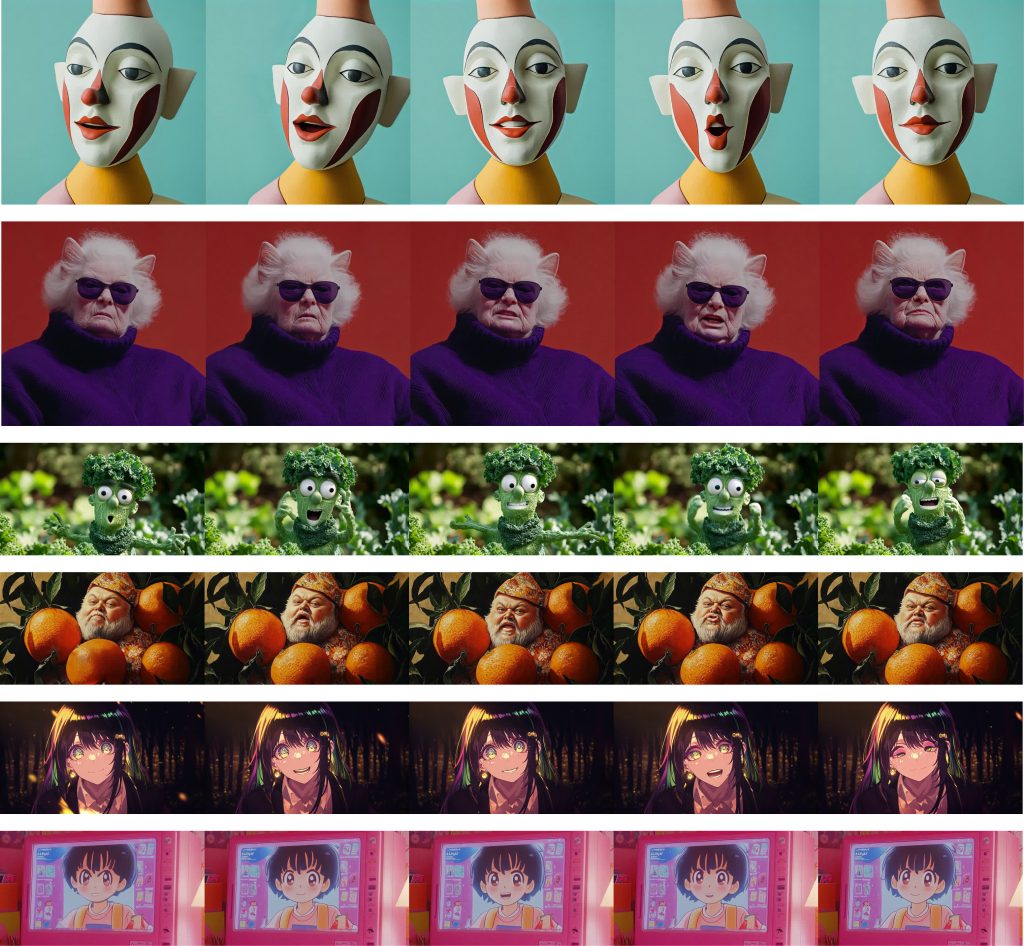
and 2D cartoon characters, and can even animate non-human images in an anthropomorphic manner.
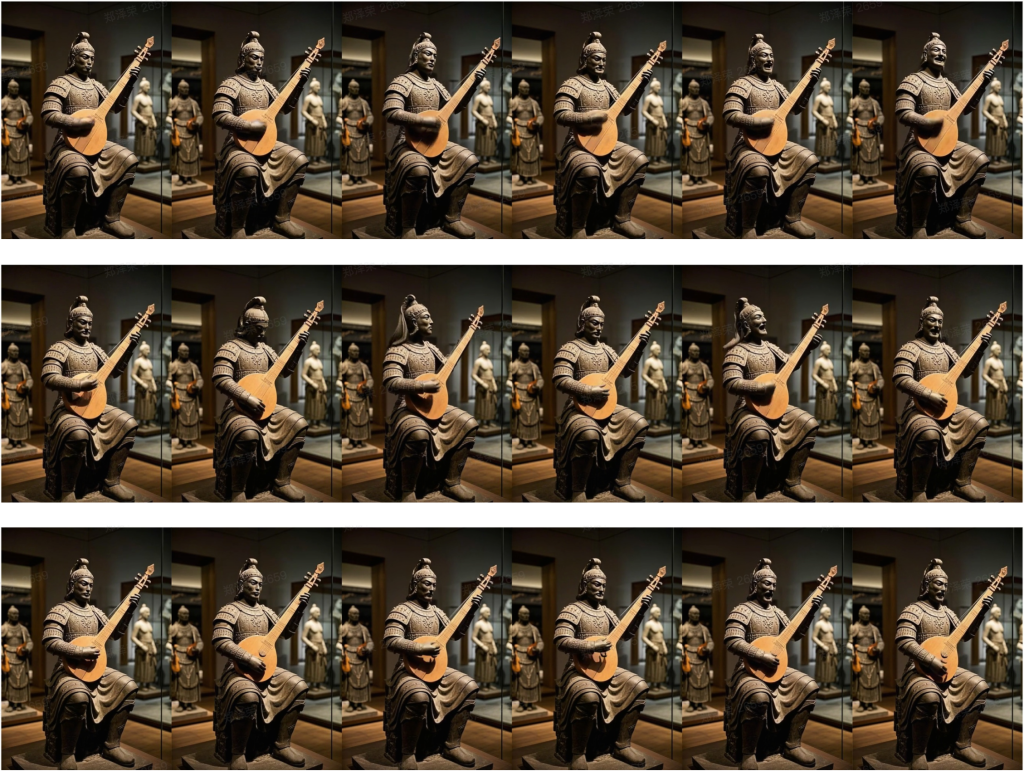
bottom: 80%) and tested in an audio-driven setting with the same input image and audio.
Abstract
End-to-end human animation, such as audio-driven talking
human generation, has undergone notable advancements in
the recent few years. However, existing methods still struggle to scale up as large general video generation models,
limiting their potential in real applications. In this paper,
we propose OmniHuman, a Diffusion Transformer-based framework that scales up data by mixing motion-related conditions into the training phase. To this end, we introduce two
training principles for these mixed conditions, along with
the corresponding model architecture and inference strategy.
These designs enable OmniHuman to fully leverage datadriven motion generation, ultimately achieving highly realistic human video generation. More importantly, OmniHuman
supports various portrait contents (face close-up, portrait,
half-body, full-body), supports both talking and singing, handles human-object interactions and challenging body poses, and accommodates different image styles. Compared to existing end-to-end audio-driven methods, OmniHuman not only
produces more realistic videos, but also offers greater flexibility in inputs. It also supports multiple driving modalities
(audio-driven, video-driven and combined driving signals).
Video samples are provided on the project page.
Add Comment