Researchers at the University of Massachusetts and the Air Force Research Laboratory Information Directorate have recently created a 3-D computing circuit that could be used to map and implement complex machine learning algorithms, such convolutional neural networks (CNNs). This 3-D circuit, presented in a paper published in Nature Electronics, comprises eight layers of memristors; electrical components that regulate the electrical current flowing in a circuit and directly implement neural network weights in hardware.
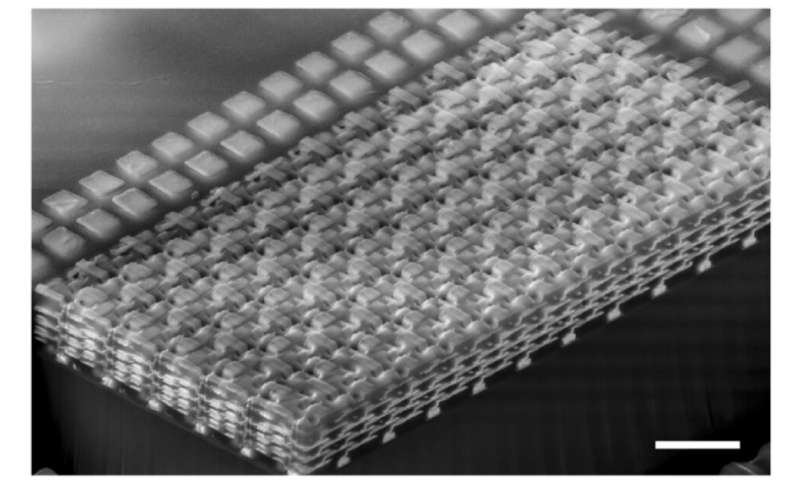
“Previously, we developed a very reliable memristive device that meets most requirements of in-memory computing for artificial neural networks, integrated the devices into large 2-D arrays and demonstrated a wide variety of machine intelligence applications,” Prof. Qiangfei Xia, one of the researchers who carried out the study, told TechXplore. “In our recent study, we decided to extend it to the third dimension, exploring the benefit of a rich connectivity in a 3-D neural network.”
Essentially, Prof. Xia and his team were able to experimentally demonstrate a 3-D computing circuit with eight memristor layers, which can all be engaged in computing processes. Their circuit differs greatly from other previously developed 3-D circuits, such as 3-D NAND flash, as these systems are usually comprised of layers with different functions (e.g. a sensor layer, a computing layer, a control layer, etc.) stacked or bonded together.
“One of the main challenges previously encountered when trying to build a multi-layer computing circuit is that there haven’t been any devices other than memristors that are stackable and yet maintain all the required performance for computing,” Peng Lin, one of the researchers who carried out the study, told Techxplore. “For example, silicon-based CMOS technology is the basic building block of the mainstream computing chips, but it is known to rely on non-stackable high-quality single crystal silicon layer and thus hard to be used for 3-D circuits.”
While memristors are excellent stacking devices, researchers have so far been unable to realize 3-D circuits with several stacked memristor layers for large-scale computing applications. In fact, building such a circuit requires highly sophisticated processes, as well as the use of techniques that can overcome challenges typically encountered when performing large-scale array operations.
“One of the prominent issues with creating a memristor array is the cell-to-cell interference, so called ‘the sneak path problem,” which originates from the passive connections between each resistor-like memristor element,” Lin said. “A 2-D memristor array can mitigate this problem by incorporating a transistor as a selecting device, but this solution cannot be applied in 3-D. As a result, the existing 3-D memristor designs, which are based on fully connected topologies, would suffer from the increasing leakages when scaled up to a large 3-D network.”
To overcome the challenges previously faced when trying to develop memristor-based 3-D circuits for large-scale computing, the researchers designed a circuit with a unique topology (i.e., arrangement of individual parts). In their circuit, memristors are linked through ‘local connections.” This means that each individual memristor only shares electrodes with a small number of devices in its vicinity. This unique design strategy leads to the suppression of most sneak paths, ultimately enabling large-scale array operations.
The unique way in which memristors are arranged and connected in the circuit devised by Prof. Xia, Lin and their colleagues also makes it ideal for implementing advanced computational techniques, such as artificial neural networks (ANNs). Past studies suggest that memristor based systems can host ANNs directly, with data flowing in their circuits and enabling the implementation of the networks’ forward/backward propagation strategies. However, almost all existing memristor arrays are arranged in regular-shaped, crossbar structures, which do not reflect the structure of ANNs.
“The fully connected topology of almost all existing memristor devices does not match the complex topologies of modern neural networks, such as CNNs, the most prominent computational techniques currently used for computer vision applications,” Lin explained. “As a result, efficient implementation of a convolutional neural network in a memristor system becomes extremely challenging.”
In contrast with previously developed circuits, the locally connected topology of the 3-D circuit designed by Prof. Xia, Lin, and their colleagues naturally matches that of CNNs, as the latter includes local connections between neurons, known as ‘local receptive fields.” This makes the circuit ideal for directly implementing complex neural networks.
“We are very proud of the successful demonstration of our 3-D memristor array with record-high eight memristor layers,” Lin said. “Although a 3-D memristor circuit of such scale and with a similar amount of stacked layers had previously been envisioned, there has not been clear evidence that such a 3-D circuit can be really built and be fully operational. Our work firmly demonstrates the capabilities of a memristor based system.”
So far, Prof. Xia, Lin and their colleagues have evaluated the effectiveness of their 3-D circuit by programming parallelly operated kernels into the memristor-array and then using the circuit to implement a CNN for pattern recognition. When running on the 3-D circuit, the CNN was found to recognize handwritten digits with a remarkable accuracy of 98%.
The researchers also successfully used the 3-D circuit to implement a technique for detecting the edges of moving objects in videos. To achieve this, they applied filters to their system’s structure, which allowed it to process different pixels simultaneously.
“We think that the unique topological design proposed in our work can open up great opportunities for designing a neuromorphic computing hardware,” Lin said. “Conventional array designs are inefficient at hosting modern neural networks because of the mismatch between the full connectivity of electrodes and the more complex connections found in neural networks. This limitation may not be the prominent issue at this initial stage of neuromorphic computing research, but it could eventually be the bottleneck to reproducing human brain-like intelligence in machines.”
Prof. Xia, Lin and their colleagues were the first to build a memristor-based 3-D computing hardware with a much higher density of computing units and advanced capabilities. Their findings suggest that such a 3-D circuit could help to mitigate the ever-growing challenges associated with scaling up modern computing devices or with using existing hardware to run advanced machine learning techniques. The researchers hope that their study will inspire additional investigations into the benefits of 3-D memristor-based circuits, ultimately prompting a shift towards design strategies that prioritize both the connectivity and functionality of computing arrays.
“We now plan to integrate the 3-D neural networks with sensor arrays so that the input fed to the neural network can be 2-D matrices, rather than 1D vectors as for most neural networks nowadays,” Prof. Xia said. “We will also process analog information directly in the integrated circuits. Research in these directions will greatly increase the throughput and power efficiency of the circuit’s information processing.”
Add Comment